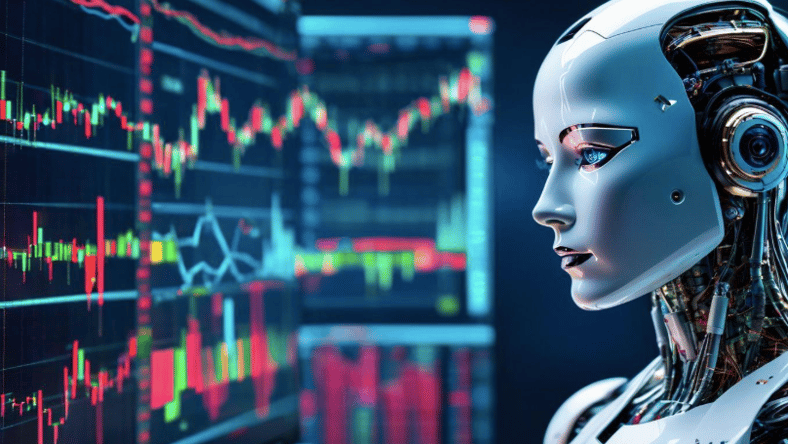
In today’s rapidly evolving financial markets, trade bots powered by artificial intelligence are revolutionizing investment decision-making by combining fundamental and sentiment analysis. This integration represents a significant advancement in automated trading, allowing for more comprehensive and nuanced market analysis than ever before. The evolution from traditional analysis methods to AI-powered approaches has created new opportunities for traders and investors to make more informed decisions based on a wider range of data points. As markets become increasingly complex, the ability to analyze multiple data sources simultaneously has become crucial for successful trading strategies.
Understanding Fundamental and Sentiment Analysis
Fundamental and sentiment analysis represent two distinct but complementary approaches to market analysis. Fundamental analysis involves evaluating a security’s intrinsic value by examining financial metrics, company performance, and market conditions. This traditional approach focuses on quantifiable data points that reflect a company’s financial health and market position. Traders and investors use this information to determine whether an asset is overvalued or undervalued relative to its current market price.
Key components of fundamental analysis include:
- Financial metrics (revenue growth, profit margins, debt levels)
- Market position and competitive advantages
- Industry trends and economic indicators
Sentiment analysis, in contrast, examines market psychology and investor emotions through various channels. This approach recognizes that market movements are not solely driven by fundamental factors but are also influenced by public perception and investor behavior. Modern AI systems can analyze vast amounts of social media activity, news coverage, and analyst opinions to gauge market sentiment and predict potential price movements.
AI Integration in Trading Analysis
Artificial intelligence systems have transformed how traders analyze and interpret market data. These sophisticated systems can simultaneously process both structured and unstructured data, creating a more comprehensive view of market conditions. The integration of AI into trading analysis has enabled traders to identify complex patterns and relationships that would be impossible to detect through traditional analysis methods.
Essential aspects of AI integration include:
- Real-time data processing from multiple sources
- Pattern recognition across different data types
- Predictive modeling using historical and current data
The power of AI lies in its ability to continuously learn and adapt to changing market conditions. As new data becomes available, AI systems update their models and adjust their strategies accordingly. This dynamic approach ensures that trading decisions are based on the most current and relevant information available.
Key Components of AI Trading Systems
Modern AI trading systems represent complex ecosystems of interconnected components that work together to analyze markets and execute trades. At their core, these systems rely on sophisticated algorithms that can process multiple data streams simultaneously while maintaining strict risk management protocols. The integration of fundamental and sentiment analysis requires carefully designed architectures that can handle both structured financial data and unstructured sentiment information.
The foundation of these systems lies in their ability to collect and process vast amounts of data in real-time. Advanced data collection modules continuously gather information from various sources, including market feeds, news outlets, social media platforms, and economic databases. This comprehensive data collection ensures that the system has access to all relevant information that might impact trading decisions.
Essential system components include:
- Data collection and preprocessing modules
- Analysis engines with machine learning capabilities
- Decision-making systems with risk management
- Execution and monitoring tools
Data Processing and Pattern Recognition
The success of AI trading systems heavily depends on their ability to process and interpret complex market data effectively. These systems employ sophisticated algorithms that can identify subtle patterns and correlations across multiple data sources. Natural Language Processing (NLP) technologies play a crucial role in analyzing textual data from news sources and social media, converting unstructured information into actionable trading signals.
Pattern recognition capabilities extend beyond simple technical analysis to identify complex market behaviors and regime changes. The systems continuously learn from new data, adapting their models to evolving market conditions. This adaptive learning process enables the AI to improve its pattern recognition capabilities over time, leading to more accurate predictions and better trading decisions.
Key technologies employed include:
- Advanced machine learning algorithms
- Neural networks for pattern recognition
- Natural Language Processing for text analysis
- Statistical modeling tools
Trading Strategy Implementation
The implementation of AI-based trading strategies requires careful consideration of multiple factors, including market conditions, risk parameters, and execution timing. These strategies must balance the insights gained from fundamental and sentiment analysis while maintaining strict risk management protocols. The system’s decision-making process considers both long-term fundamental factors and short-term sentiment indicators to generate trading signals.
Strategy execution involves sophisticated algorithms that can determine optimal entry and exit points while managing position sizes and risk exposure. These algorithms take into account market liquidity, potential impact on price, and transaction costs to ensure efficient execution of trades. The system continuously monitors market conditions and adjusts its strategies accordingly, ensuring adaptability to changing market environments.
Risk Management and Optimization
Effective risk management forms the cornerstone of successful AI trading systems. These systems employ multiple layers of risk controls to protect against adverse market movements and system failures. Risk management protocols operate continuously, monitoring position sizes, exposure levels, and market volatility to maintain risk within acceptable parameters.
Critical risk management elements:
- • Position size limitations
- • Volatility-based adjustments
- • Correlation analysis
- • Performance monitoring
The optimization process involves continuous testing and refinement of trading strategies based on historical and real-time data. This includes regular backtesting of strategies, parameter optimization, and performance evaluation against various market conditions. The system’s ability to adapt and optimize its strategies ensures long-term sustainability and consistent performance in varying market environments.
By combining fundamental and sentiment analysis within a robust AI framework, these systems offer traders and investors powerful tools for navigating complex financial markets. The integration of multiple analysis methods, coupled with sophisticated risk management and optimization protocols, represents the cutting edge of modern trading technology.
Future Implications and Market Impact
The integration of AI-powered fundamental and sentiment analysis is fundamentally changing the landscape of financial markets. As these systems become more sophisticated, we’re witnessing a shift in how market participants approach trading decisions. The ability to process and analyze vast amounts of data in real-time is creating new opportunities while also raising important questions about market efficiency and fairness.
The impact of these systems extends beyond individual trading performance to influence overall market dynamics. Institutional investors and large trading firms are increasingly relying on AI-powered analysis to gain competitive advantages. This trend is likely to continue as technology advances and becomes more accessible to a broader range of market participants.
Key market impacts include:
- Enhanced market efficiency
- Increased trading volume
- Improved price discovery
- Reduced market manipulation risks
Challenges and Considerations
Despite the significant advantages offered by AI trading systems, several challenges and considerations must be addressed. Data quality remains a critical concern, as the effectiveness of AI analysis depends heavily on the accuracy and reliability of input data. Market participants must carefully validate data sources and implement robust quality control measures to ensure reliable analysis.
Another significant challenge lies in maintaining system stability during periods of market stress. AI systems must be designed with sufficient redundancy and fail-safes to prevent cascading failures during extreme market conditions. Additionally, the complexity of these systems requires sophisticated monitoring and maintenance protocols to ensure consistent performance.
Important considerations include:
- Data quality and reliability
- System stability and redundancy
- Regulatory compliance
- Ethical implications
Best Practices and Implementation Guidelines
Successful implementation of AI-powered trading systems requires careful attention to best practices and industry standards. Organizations must develop comprehensive frameworks for system development, testing, and deployment. This includes establishing clear protocols for risk management, compliance monitoring, and performance evaluation.
Regular system maintenance and updates are essential to ensure optimal performance and adaptation to changing market conditions. Organizations should maintain detailed documentation of system architecture, trading strategies, and risk management protocols. Regular training and education for system operators and supervisors help ensure proper system oversight and management.
The future success of AI-powered trading systems will depend on their ability to adapt to evolving market conditions while maintaining robust risk management and compliance standards. As these systems continue to evolve, market participants must stay informed about technological advances and regulatory changes that may impact their operations.
Concluding Thoughts
The combination of fundamental and sentiment analysis through AI represents a significant advancement in trading technology. These systems offer powerful tools for market analysis and decision-making, but their successful implementation requires careful attention to various technical and operational factors. As the technology continues to evolve, we can expect to see further innovations in how AI is used to analyze and trade in financial markets.
Success factors for implementation:
- Robust system architecture
- Comprehensive testing protocols
- Regular maintenance and updates
- Continuous monitoring and optimization
The future of trading will likely see increased adoption of AI-powered analysis tools, making it essential for market participants to understand and adapt to these technological advances. Organizations that successfully implement these systems while maintaining strong risk management and compliance standards will be well-positioned to benefit from the opportunities they present.